The papers below discuss some uses of PROCESS as well as describe some features or "hacks" not documented in Introduction to Mediation, Moderation, and Conditional Process Analysis.

Hayes, A. F., Allison, P. D., & Alexander, S. M. (2024). Errors-in-variables regression as a viable approach to mediation analysis with random error-tainted measurements: Estimation, effectiveness, and an easy-to-use implementation. Manuscript in review.
Mediation analysis, popular in many disciplines that rely on behavioral science data analysis techniques, is often conducted using ordinary least squares (OLS) regression analysis methods. Given that one of OLS regression’s greatest weaknesses is its susceptibility to estimation bias that results from unaccounted-for random measurement error in variables on the right-hand sides of the equation, many published mediation analyses certainly contain some and perhaps substantial bias in the direct, indirect, and total effects. In this manuscript, we offer errors-in-variables (EIV) regression as an easy-to-use alternative to OLS regression when a researcher has reasonable estimates of the reliability of the variables in the analysis. In three real-data examples, we show that EIV regression-based mediation analysis produces estimates that are largely equivalent to those obtained using an alternative, more analytically complex approach that accounts for measurement error—single-indicator latent variable structural equation modeling—yet quite different from the results generated by standard OLS regression that ignores random measurement error. In a small-scale simulation, we also establish that EIV regression successfully recovers the parameters of a mediation model involving variables adulterated by random measurement error while OLS regression generates biased estimates. To facilitate the adoption of EIV regression, we describe an implementation in version 5 of the PROCESS macro for SPSS, SAS, and R that we believe now eliminates most any excuse one can conjure for not accounting for random measurement error when conducting a mediation analysis.
Mediation analysis, popular in many disciplines that rely on behavioral science data analysis techniques, is often conducted using ordinary least squares (OLS) regression analysis methods. Given that one of OLS regression’s greatest weaknesses is its susceptibility to estimation bias that results from unaccounted-for random measurement error in variables on the right-hand sides of the equation, many published mediation analyses certainly contain some and perhaps substantial bias in the direct, indirect, and total effects. In this manuscript, we offer errors-in-variables (EIV) regression as an easy-to-use alternative to OLS regression when a researcher has reasonable estimates of the reliability of the variables in the analysis. In three real-data examples, we show that EIV regression-based mediation analysis produces estimates that are largely equivalent to those obtained using an alternative, more analytically complex approach that accounts for measurement error—single-indicator latent variable structural equation modeling—yet quite different from the results generated by standard OLS regression that ignores random measurement error. In a small-scale simulation, we also establish that EIV regression successfully recovers the parameters of a mediation model involving variables adulterated by random measurement error while OLS regression generates biased estimates. To facilitate the adoption of EIV regression, we describe an implementation in version 5 of the PROCESS macro for SPSS, SAS, and R that we believe now eliminates most any excuse one can conjure for not accounting for random measurement error when conducting a mediation analysis.

Coutts, J. J., & Hayes, A. F. (2023). Questions of value, questions of magnitude: An exploration and application of methods for comparing indirect effects in multiple mediator models. Behavior Research Methods, 55, 3772-3785.
Mediation analysis is widely used to test and inform theory and debate about the mechanism(s) by which causal effects operate, quantitatively operationalized as an indirect effect in a mediation model. Most effects operate through multiple mechanisms simultaneously, and a mediation model is likely more realistic when it is specified to capture multiple mechanisms at the same time with the inclusion of more than one mediator in the model. This also allows an investigator to compare indirect effects to each other. After introducing the mechanics of mediation analysis, we advocate formally comparing indirect effects in models that include more than one mediator, focusing on the important distinction between questions and claims about value (i.e., Are two indirect effects the same number?) versus magnitude (i.e., Are two indirect effects equidistant from zero or the same strength?). After discussing the shortcomings of the conventional method for comparing two indirect effects in a multiple mediator model—which only answers a question about magnitude in some circumstances—we introduce several methods that—unlike the conventional approach—always answer questions about difference in magnitude. We illustrate the use of these methods and provide code that implements them in popular software. We end by summarizing simulation findings and recommend which method(s) to prefer and when.
Mediation analysis is widely used to test and inform theory and debate about the mechanism(s) by which causal effects operate, quantitatively operationalized as an indirect effect in a mediation model. Most effects operate through multiple mechanisms simultaneously, and a mediation model is likely more realistic when it is specified to capture multiple mechanisms at the same time with the inclusion of more than one mediator in the model. This also allows an investigator to compare indirect effects to each other. After introducing the mechanics of mediation analysis, we advocate formally comparing indirect effects in models that include more than one mediator, focusing on the important distinction between questions and claims about value (i.e., Are two indirect effects the same number?) versus magnitude (i.e., Are two indirect effects equidistant from zero or the same strength?). After discussing the shortcomings of the conventional method for comparing two indirect effects in a multiple mediator model—which only answers a question about magnitude in some circumstances—we introduce several methods that—unlike the conventional approach—always answer questions about difference in magnitude. We illustrate the use of these methods and provide code that implements them in popular software. We end by summarizing simulation findings and recommend which method(s) to prefer and when.
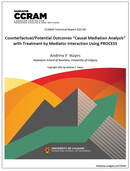
Counterfactual/potential outcomes "causal mediation analysis" with treatment by mediator interaction using PROCESS. Canadian Centre for Research Analysis and Methods Technical Report
Researchers typically assume no interaction between X and M in a mediation model. With the release of version 4.2 of PROCESS in October 2022, PROCESS can estimate mediation models (model 4 only) that allow for interaction between X and M. The computations follow the counterfactual/potential outcomes tradition, resulting in counterfactually defined natural indirect, natural direct, and controlled direct effects of X. This technical report describes how to tell PROCESS to allow for X by M interaction in a mediation model and documents various options available in release 4.2 for counterfactual mediation analysis.
Researchers typically assume no interaction between X and M in a mediation model. With the release of version 4.2 of PROCESS in October 2022, PROCESS can estimate mediation models (model 4 only) that allow for interaction between X and M. The computations follow the counterfactual/potential outcomes tradition, resulting in counterfactually defined natural indirect, natural direct, and controlled direct effects of X. This technical report describes how to tell PROCESS to allow for X by M interaction in a mediation model and documents various options available in release 4.2 for counterfactual mediation analysis.
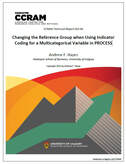
Changing the reference group when using indicator coding for a multicategorical variable in PROCESS. Canadian Centre for Research Analysis and Methods Technical Report
By default, PROCESS will use the category with the numerically smallest code in the data as the reference group when a variable is specified as multicategorical. This paper addresses some options available for changing the reference group, including recoding of the categories in the data and using the xcatcode option for creating your own coding system.
By default, PROCESS will use the category with the numerically smallest code in the data as the reference group when a variable is specified as multicategorical. This paper addresses some options available for changing the reference group, including recoding of the categories in the data and using the xcatcode option for creating your own coding system.
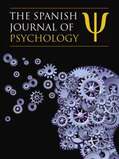
Igartua, J.-J. & Hayes, A. F. (2021). Mediation, moderation, and conditional process analysis: Concepts, computations, and some common confusions. Spanish Journal of Psychology, 24, e49.
This work provides a conceptual introduction to mediation, moderation, and conditional process analysis in psychological research. We discuss the concepts of direct effect, indirect effect, total effect, conditional effect, conditional direct effect, conditional indirect effect, and the index of moderated mediation, while providing our perspective on certain analysis and interpretation confusions that sometimes arise in practice in this journal and elsewhere, such as reliance on the causal steps approach and the Sobel test in mediation analysis, misinterpreting the regression coefficients in a model that includes a product of variables, and subgroups mediation analysis rather than conditional process analysis when exploring whether an indirect effect depends on a moderator. We also illustrate how to conduct various analyses that are the focus of this paper with the freely-available PROCESS procedure available for SPSS, SAS, and R, using data from an experimental investigation on the effectiveness of personal or testimonial narrative messages in improving intergroup attitudes.
This work provides a conceptual introduction to mediation, moderation, and conditional process analysis in psychological research. We discuss the concepts of direct effect, indirect effect, total effect, conditional effect, conditional direct effect, conditional indirect effect, and the index of moderated mediation, while providing our perspective on certain analysis and interpretation confusions that sometimes arise in practice in this journal and elsewhere, such as reliance on the causal steps approach and the Sobel test in mediation analysis, misinterpreting the regression coefficients in a model that includes a product of variables, and subgroups mediation analysis rather than conditional process analysis when exploring whether an indirect effect depends on a moderator. We also illustrate how to conduct various analyses that are the focus of this paper with the freely-available PROCESS procedure available for SPSS, SAS, and R, using data from an experimental investigation on the effectiveness of personal or testimonial narrative messages in improving intergroup attitudes.
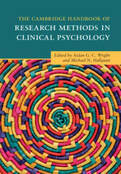
Rockwood, N. J., & Hayes, A. F. (2020). Mediation, moderation, and conditional process analysis: Regression-based approaches for clinical research. In A. G. C. Wright and M. N. Hallquist (Eds.) Handbook of research methods in clinical psychology. Cambridge University Press.
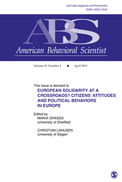
Hayes, A. F., & Rockwood, N. J. (2020). Conditional process analysis: Concepts, computation, and advances in the modeling of the contingencies of mechanisms. American Behavioral Scientist, 64, 19-54.
Behavioral scientists use mediation analysis to understand the mechanism(s) by which an effect operates and moderation analysis to understand the contingencies or boundary conditions of effects. Yet how effects operate (i.e., the mechanism at work) and their boundary conditions (when they occur) are not necessarily independent, though they are often treated as such. Conditional process analysis is an analytical strategy that integrates mediation and moderation analysis with the goal of examining and testing hypotheses about how mechanisms vary as a function of context or individual differences. In this paper, we provide a conceptual primer on conditional process analysis for those not familiar with the integration of moderation and mediation analysis, while also describing some recent advances and innovations for the more experienced conditional process analyst. After overviewing fundamental modeling principles using ordinary least squares regression, we discuss the extension of these fundamentals to models with more than one mediator and more than one moderator. We describe a differential dominance conditional process model and overview the concepts of partial, conditional, and moderated moderated mediation. We also discuss multilevel conditional process analysis and comment on implementation of conditional process analysis in statistical computing software.
Behavioral scientists use mediation analysis to understand the mechanism(s) by which an effect operates and moderation analysis to understand the contingencies or boundary conditions of effects. Yet how effects operate (i.e., the mechanism at work) and their boundary conditions (when they occur) are not necessarily independent, though they are often treated as such. Conditional process analysis is an analytical strategy that integrates mediation and moderation analysis with the goal of examining and testing hypotheses about how mechanisms vary as a function of context or individual differences. In this paper, we provide a conceptual primer on conditional process analysis for those not familiar with the integration of moderation and mediation analysis, while also describing some recent advances and innovations for the more experienced conditional process analyst. After overviewing fundamental modeling principles using ordinary least squares regression, we discuss the extension of these fundamentals to models with more than one mediator and more than one moderator. We describe a differential dominance conditional process model and overview the concepts of partial, conditional, and moderated moderated mediation. We also discuss multilevel conditional process analysis and comment on implementation of conditional process analysis in statistical computing software.
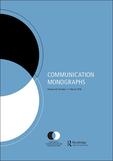
Hayes, A. F. (2018). Partial, conditional, and moderated moderated mediation: Quantification, inference, and interpretation. Communication Monographs, 85, 4-40.
Mediation of variable X’s effect on outcome Y through a mediator M is said to be moderated if the indirect effect of X on Y through M depends on another variable. Hayes (2015) introduced a simple approach to testing a linear moderated mediation hypothesis based on an index of moderated mediation—the weight for the moderator in a linear function relating the size of the indirect effect to the moderator. In this article, I extend this approach to mediation models with more than one moderator. I introduce relevant concepts and an inferential approach to testing if X’s indirect effect on Y through M is moderated by one variable when a second proposed moderator is held constant (partial moderated mediation), conditioned on a second variable (conditional moderated mediation), or is dependent on a second variable (moderated moderated mediation). I provide examples of the analysis, discuss model visualization, and illustrate implementation in the PROCESS macro for SPSS and SAS.
Mediation of variable X’s effect on outcome Y through a mediator M is said to be moderated if the indirect effect of X on Y through M depends on another variable. Hayes (2015) introduced a simple approach to testing a linear moderated mediation hypothesis based on an index of moderated mediation—the weight for the moderator in a linear function relating the size of the indirect effect to the moderator. In this article, I extend this approach to mediation models with more than one moderator. I introduce relevant concepts and an inferential approach to testing if X’s indirect effect on Y through M is moderated by one variable when a second proposed moderator is held constant (partial moderated mediation), conditioned on a second variable (conditional moderated mediation), or is dependent on a second variable (moderated moderated mediation). I provide examples of the analysis, discuss model visualization, and illustrate implementation in the PROCESS macro for SPSS and SAS.

Hayes, A. F., Montoya, A. K., & Rockwood, N. J. (2017). The analysis of mechanisms and their contingencies: PROCESS versus structural equation modeling. Australasian Marketing Journal, 25, 76-81.
Marketing, consumer, and organizational behavior researchers interested in studying the mechanisms by which effects operate and the conditions that enhance or inhibit such effects often rely on statistical mediation and conditional process analysis (also known as the analysis of "moderated mediation"). Model estimation is typically done with ordinary least squares regression-based path analysis, such as implemented in the popular PROCESS macro for SPSS and SAS (Hayes, 2013), or using a structural equation modeling program. In this article, we answer a few frequently-asked questions about the difference between PROCESS and structural equation modeling and show by way of example that for observed variable models, the choice of which to use is inconsequential, as the results are largely identical. We end by discussing considerations to ponder when making the choice between PROCESS and structural equation modeling.
Marketing, consumer, and organizational behavior researchers interested in studying the mechanisms by which effects operate and the conditions that enhance or inhibit such effects often rely on statistical mediation and conditional process analysis (also known as the analysis of "moderated mediation"). Model estimation is typically done with ordinary least squares regression-based path analysis, such as implemented in the popular PROCESS macro for SPSS and SAS (Hayes, 2013), or using a structural equation modeling program. In this article, we answer a few frequently-asked questions about the difference between PROCESS and structural equation modeling and show by way of example that for observed variable models, the choice of which to use is inconsequential, as the results are largely identical. We end by discussing considerations to ponder when making the choice between PROCESS and structural equation modeling.
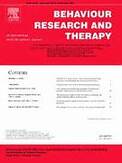
Hayes, A. F., & Rockwood, N. J. (2017). Regression-based statistical mediation and moderation analysis in clinical research: Observations, recommendations, and implementation. Behaviour Research and Therapy, 98, 39-57. [data]
There have been numerous treatments in the clinical research literature about various design, analysis, and interpretation considerations when testing hypotheses about mechanisms and contingencies of effects, popularly known as mediation and moderation analysis. In this paper we address the practice of mediation and moderation analysis using linear regression in the pages of Behaviour Research and Therapy and offer some observations and recommendations, debunk some popular myths, describe some new advances, and provide an example of mediation, moderation, and their integration as conditional process analysis using the PROCESS macro for SPSS and SAS. Our goal is to nudge clinical researchers away from historically significant but increasingly old school approaches toward modifications, revisions, and extensions that characterize more modern thinking about the analysis of the mechanisms and contingencies of effects.
There have been numerous treatments in the clinical research literature about various design, analysis, and interpretation considerations when testing hypotheses about mechanisms and contingencies of effects, popularly known as mediation and moderation analysis. In this paper we address the practice of mediation and moderation analysis using linear regression in the pages of Behaviour Research and Therapy and offer some observations and recommendations, debunk some popular myths, describe some new advances, and provide an example of mediation, moderation, and their integration as conditional process analysis using the PROCESS macro for SPSS and SAS. Our goal is to nudge clinical researchers away from historically significant but increasingly old school approaches toward modifications, revisions, and extensions that characterize more modern thinking about the analysis of the mechanisms and contingencies of effects.
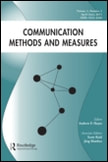
Hayes, A. F., & Montoya, A. K. (2017). A tutorial on testing, visualizing, and probing an interaction involving a multicategorical variable in linear regression analysis. Communication Methods and Measures, 11, 1-30. [data]
Empirical communication scholars and scientists in other fields regularly use regression models to test moderation hypotheses. When the independent variable X and moderator M are dichotomous or continuous, the practice of testing a linear moderation hypothesis using regression analysis by including the product of X and M in a model of dependent variable Y is widespread. But many research designs include multicategorical independent variables or moderators, such as in an experiment with three or more versions of a stimulus where participants are randomly assigned to one of them. Researchers are less likely to receive training about how to properly test a moderation hypothesis using regression analysis in such a situation. In this tutorial, we describe how to test, visualize, and probe interactions involving a multicategorical variable using linear regression analysis. While presenting and discussing the fundamentals—fundamentals that are not software specific—we emphasize the use of the PROCESS macro for SPSS and SAS, as it greatly simplifies the computations and potential for error that exists when doing computations by hand or using spreadsheets based on formulas in existing books on this topic. We also introduce an iterative computational implementation of the Johnson-Neyman technique for finding regions of significance of the effect of a multicategorical independent variable when the moderator is continuous.
Empirical communication scholars and scientists in other fields regularly use regression models to test moderation hypotheses. When the independent variable X and moderator M are dichotomous or continuous, the practice of testing a linear moderation hypothesis using regression analysis by including the product of X and M in a model of dependent variable Y is widespread. But many research designs include multicategorical independent variables or moderators, such as in an experiment with three or more versions of a stimulus where participants are randomly assigned to one of them. Researchers are less likely to receive training about how to properly test a moderation hypothesis using regression analysis in such a situation. In this tutorial, we describe how to test, visualize, and probe interactions involving a multicategorical variable using linear regression analysis. While presenting and discussing the fundamentals—fundamentals that are not software specific—we emphasize the use of the PROCESS macro for SPSS and SAS, as it greatly simplifies the computations and potential for error that exists when doing computations by hand or using spreadsheets based on formulas in existing books on this topic. We also introduce an iterative computational implementation of the Johnson-Neyman technique for finding regions of significance of the effect of a multicategorical independent variable when the moderator is continuous.
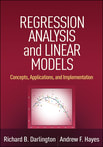
Darlington, R. B., & Hayes, A. F. (2017). Regression analysis and linear models: Concepts, applications, and implementation. New York: The Guilford Press.
This book contains two chapters on moderation and one on mediation. The chapter on mediation analysis includes an example using PROCESS.
This book contains two chapters on moderation and one on mediation. The chapter on mediation analysis includes an example using PROCESS.
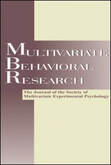
Hayes, A. F. (2015). An index and test of linear moderated mediation. Multivariate Behavioral Research, 50, 1-22.
I describe a test of linear moderated mediation in path analysis based on an interval estimate of the parameter of a function linking the indirect effect to values of a moderator---a parameter that I call the index of moderated mediation. This test can be used for models that integrate moderation and mediation in which the relationship between the indirect effect and the moderator is estimated as linear, including many of the models described by Edwards and Lambert (2007} and Preacher, Rucker, and Hayes (2007} as well as extensions of these models to processes involving multiple mediators operating in parallel or in serial. Generalization of the method to latent variable models is straightforward. Three empirical examples describe the computation of the index and the test, and its implementation is illustrated using Mplus and the PROCESS macro for SPSS and SAS.
NOTE: PROCESS v3 syntax is different than PROCESS v2. In addition, PROCESS v3 can now estimate moderated serial mediation models, eliminating the need for the use of the trick described in the appendix of this article. See the PROCESS v3 supplement for information.
I describe a test of linear moderated mediation in path analysis based on an interval estimate of the parameter of a function linking the indirect effect to values of a moderator---a parameter that I call the index of moderated mediation. This test can be used for models that integrate moderation and mediation in which the relationship between the indirect effect and the moderator is estimated as linear, including many of the models described by Edwards and Lambert (2007} and Preacher, Rucker, and Hayes (2007} as well as extensions of these models to processes involving multiple mediators operating in parallel or in serial. Generalization of the method to latent variable models is straightforward. Three empirical examples describe the computation of the index and the test, and its implementation is illustrated using Mplus and the PROCESS macro for SPSS and SAS.
NOTE: PROCESS v3 syntax is different than PROCESS v2. In addition, PROCESS v3 can now estimate moderated serial mediation models, eliminating the need for the use of the trick described in the appendix of this article. See the PROCESS v3 supplement for information.
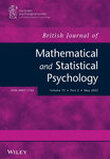
Hayes, A. F., & Preacher, K. J. (2014). Statistical mediation analysis with a multicategorical independent variable. British Journal of Mathematical and Statistical Psychology, 67, 451-470.
Virtually all discussions and applications of statistical mediation analysis have been based on the condition that the independent variable is dichotomous or continuous, even though investigators frequently are interested in testing mediation hypotheses involving a multicategorical independent variable (such as two or more experimental conditions relative to a control group). We provide a tutorial illustrating an approach to estimation of and inference about direct, indirect, and total effects in statistical mediation analysis with a multicategorical independent variable. The approach is mathematically equivalent to analysis of (co)variance and reproduces the observed and adjusted group means while also generating effects having simple interpretations. Supplementary material available online includes extensions to this approach and Mplus, SPSS, and SAS code that implements it. [Download online supplement]
Virtually all discussions and applications of statistical mediation analysis have been based on the condition that the independent variable is dichotomous or continuous, even though investigators frequently are interested in testing mediation hypotheses involving a multicategorical independent variable (such as two or more experimental conditions relative to a control group). We provide a tutorial illustrating an approach to estimation of and inference about direct, indirect, and total effects in statistical mediation analysis with a multicategorical independent variable. The approach is mathematically equivalent to analysis of (co)variance and reproduces the observed and adjusted group means while also generating effects having simple interpretations. Supplementary material available online includes extensions to this approach and Mplus, SPSS, and SAS code that implements it. [Download online supplement]
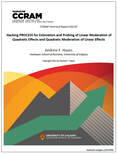
Hacking PROCESS for estimation and probing of linear moderation of quadratic effects and quadratic moderation of linear effects. Canadian Centre for Research Analysis and Methods Technical Report.
PROCESS model 1, used for estimating, testing, and probing interactions in ordinary least squares and logistic regression, constrains focal predictor X's linear effect on outcome variable Y to be linearly moderated by a single moderator M. In this document I describe how to hack PROCESS to get it to estimate a model that includes linear moderation by M of a quadratic effect of X on Y, and quadratic moderation by M of a linear effect of X on Y. Instructions are provided for the implementation of the pick-a-point and Johnson-Neyman techniques for probing interactions in models that combine quadratic nonlinearity and moderation.
PROCESS model 1, used for estimating, testing, and probing interactions in ordinary least squares and logistic regression, constrains focal predictor X's linear effect on outcome variable Y to be linearly moderated by a single moderator M. In this document I describe how to hack PROCESS to get it to estimate a model that includes linear moderation by M of a quadratic effect of X on Y, and quadratic moderation by M of a linear effect of X on Y. Instructions are provided for the implementation of the pick-a-point and Johnson-Neyman techniques for probing interactions in models that combine quadratic nonlinearity and moderation.